How to build a churn prediction model that actually works
21 December 2020
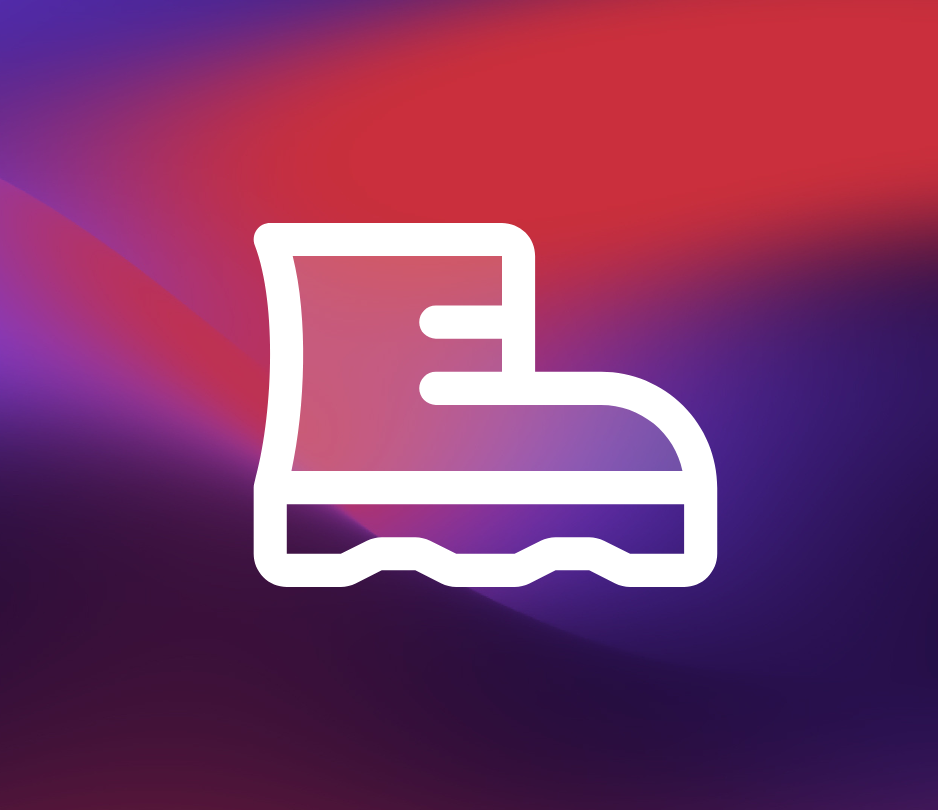
Increasing customer retention is one of the biggest challenges subscription businesses and retailers are facing. While there are a number of things you can do to improve retention rates, predicting customers at risk of leaving and changing their minds is one of the most cost-efficient ways to do so. That’s why customer churn predictions became a hot topic.
Predicting churn is not the hard part
The truth is, churn prediction is easy. ‘Hold my beer’ while I’m implementing this machine learning model...
As a data scientist it is super cool to show off with your machine learning or artificial intelligence superpowers. But how many times did it happen to you, dear data scientist, that the model you produced was never used by the business? Too many times, I know.
And how many times did it happen to you, dear marketer, that a fancy machine learning model didn’t answer your business needs? Too many times, I know.
The solution? A table to put both your beers on… You’ll need both your business knowledge and data science magic together to build an actionable churn prediction model that allows you to effectively retain customers.
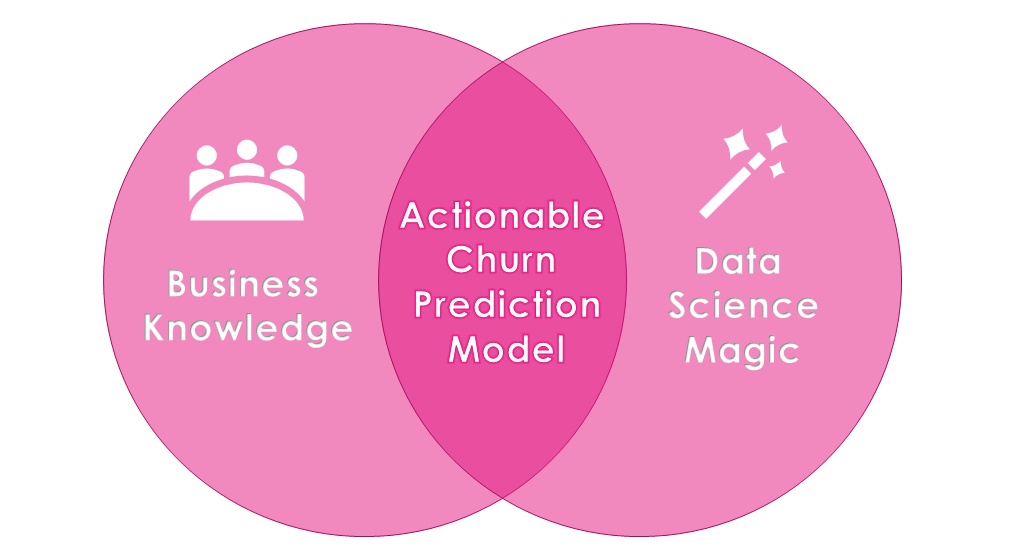
Sound a bit fluffy? I know. Let’s have a closer look.
One does not simply define churn!
The first step in building a churn prediction model is labeling customers as churners and non-churners. Easy-peasy! So, what’s the definition of customer churn?
A churner is defined as a customer who ends his relationship with your company.
This sounds just as easy as switching your relationship status on Facebook. But if you want to create real impact with your churn prediction model, ‘it’s complicated’ is better suited here. You’ll need to define churn in an actionable way.
These are the 3 key aspects to make your churn definition actionable.
- Distinguish financial churn data from commercial churn data. Financial churners are customers who aren’t properly paying you for the services/products they use. It’s clear you’re better off without this type of customers. People deciding themselves to end the relationship with your company are commercial churners. These customers are valuable for your company and you want to retain them.
- Second, defining the end of a relationship depends heavily on your industry. In subscription businesses like SaaS or telecom, churn can be identified when someone is cancelling the subscription. However, identifying such a breaking point is impossible in a retail business. For a retailer or e-commerce business, an extensive analysis on patterns in buying behavior combined with business expertise is needed in order to define and identify churn, and in that way your churn rate.
- Finally, incorporate a time window in your definition of churn. A model that predicts that a customer will churn tomorrow, might be very accurate, but it doesn’t give you ample time to take actions. Therefore, you need to define upfront how much time is needed to establish your retainment actions. On the other hand, predicting that a customer will churn in 1 year is much more difficult. This makes for a real balancing exercise between time needed to take action and the accuracy of your predictions.
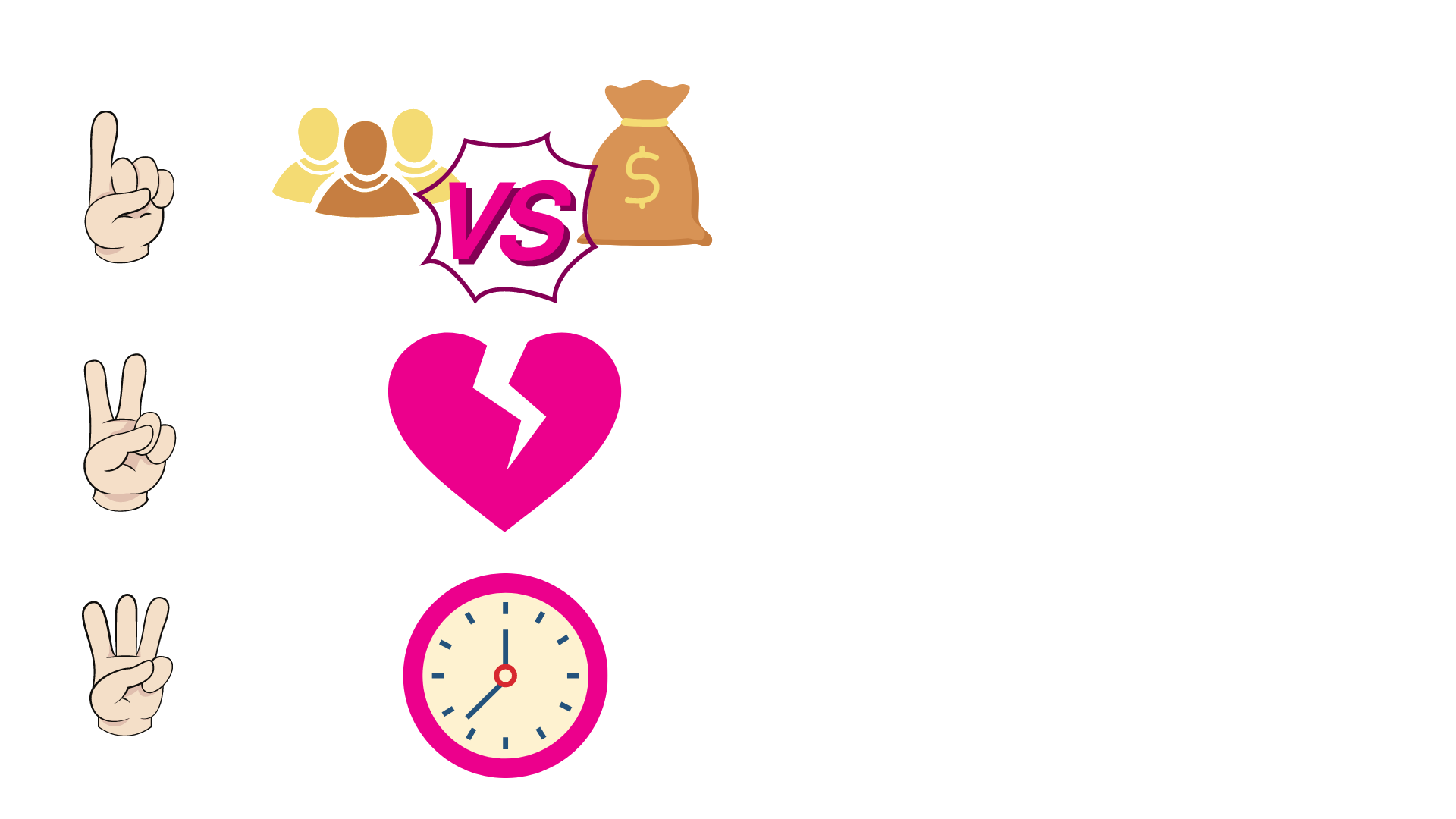
Please feed me! Feature engineering and data leakage on the menu.
As previously said, building a prediction model is not a big deal. Really. As long as you feed your model the right data.
Typical data to feed your churn prediction model includes customer data, transactional data and usage data.
All these data sources (and many more) contain valuable information to make accurate predictions. However, the real value for your prediction model lies in transforming these static variables into features that are able to grasp change.
It rarely happens that a customer decides to churn out of the blue. Churn is usually a gradual process, so it’s important to feed your prediction model with features that grab actual change in behavior, preceding the actual breaking point. That is why churn analysis is key.
It rarely happens that a customer decides to churn out of the blue. Churn is usually a gradual process.
Next to that, if you want to plug in retention campaigns, you need to train a model that reaches the same accuracy on new, unseen data. If this is not the case, you probably fell victim to data leakage. This occurs when your dataset contains information that is directly linked to churn. An example of telecom customer churn prediction, a customer calls to customer service in order to announce he will stop his subscription next month. A customer support agent encodes this in the CRM system. When incorporating this kind of data while training a model, you end up with accuracy scores that are through the roof. In reality, however, you’ll never be able to replicate these results.
And oh please, keep track of your past campaigns and exclude previously targeted customers from your input data will you? Imagine you targeted a customer highly likely to churn (Hooray, nice prediction!) and your campaign worked as a charm (High five, marketeers!). Since you were able to retain this customer, he will be labelled as non-churner in your data set. By giving the data of this customer to your magic prediction model, your model will link the highly alarming churn signals to the non-churner. Take your time when doing customer churn analysis.
May I have your retention please! Personalized marketing to the rescue!
Cool! You’ve built an accurate model that identifies customers likely to churn within a certain time frame. But now you need to find a way to retain them? Giving everyone a € 20 discount is probably not affordable and a bad idea overall.
This point is not the end of the data science job. It’s also not the start of the marketing job. You have to fight this fight together!
If you want your retention campaigns to be as effective as possible, it’s crucial to understand why a customer is at risk. And that dear friends, is the cherry on the cake you were looking for. It’s the key information that will allow you to be relevant and to turn someone likely to churn into a loyal customer.
To maximize the effectiveness of your retention campaigns, a relevant intervention is the key. But to be relevant to each customer, it’s crucial to understand why a customer is at risk.
If you want your retention campaigns to be as effective as possible, it’s crucial to understand why a customer is at risk. Never lose a root cause customer analysis out of sight.
Imagine for instance that you’re totally fed up with your telecom provider because of recurring network issues. Calling them with a discount will be like throwing gas on a fire. A friendly customer support agent pro-actively offering you a solution for your network problems on the other hand, will work like a charm!
So on top of a churn prediction model that tells you who is at risk, you now have a model that identifies the churn root cause for every customer.
How to identify the root cause of a customer at risk?
There are two ways to do this. The first approach is to start from your prediction model and investigate which features are most important in predicting churn at a customer level. Cluster these features into meaningful categories and see which ones are on top of the ranking.
The second approach is to start from business knowledge about reasons to churn and translate this into measurable data dimensions. Score each customer on each dimension and see where each customer is standing out the other ones.
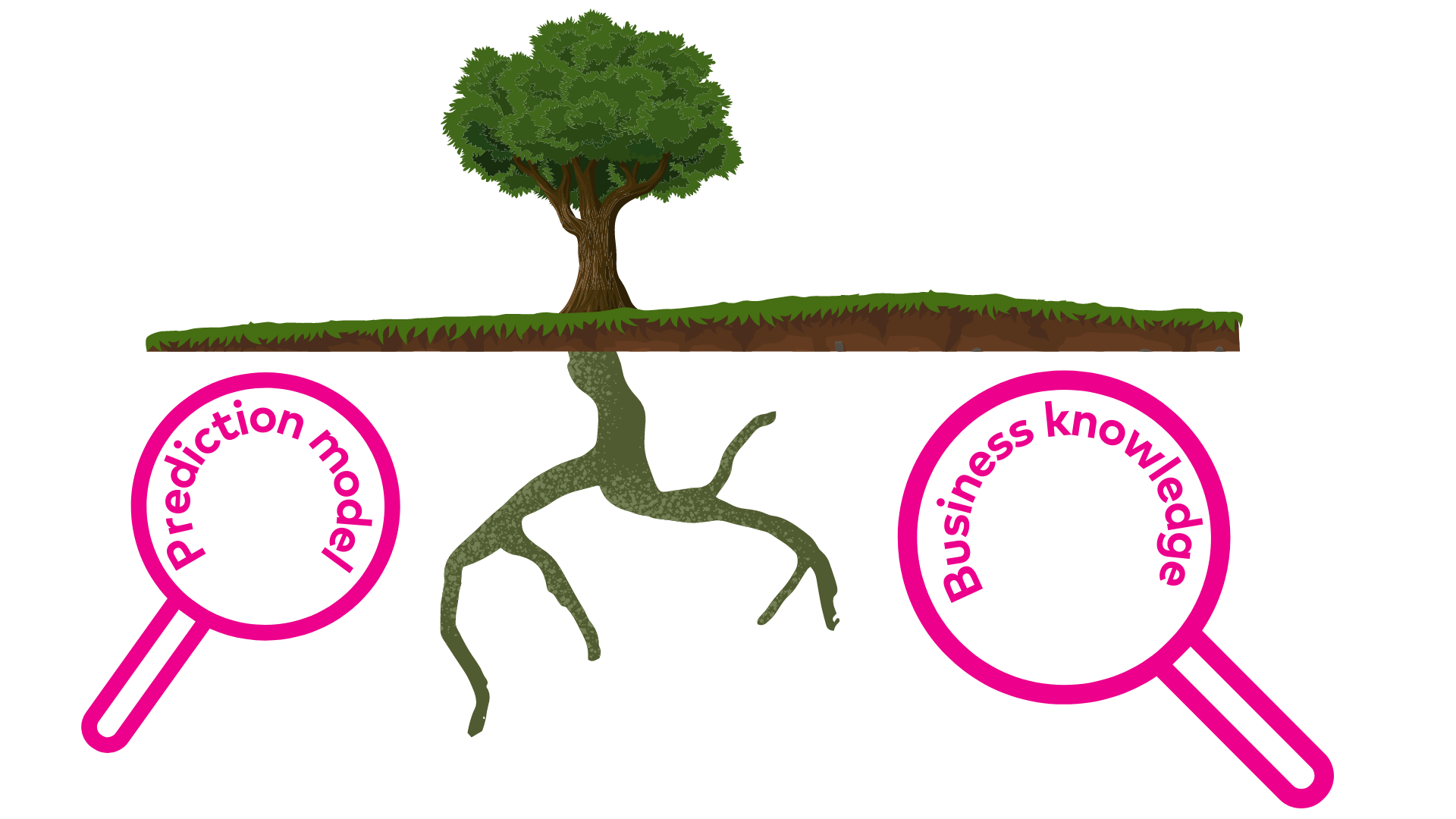
Finally, link a relevant marketing action to each reason for churn, and here you go!
Are you looking to build a prediction model for your business? Having trouble making it actionable? Hopefully this article helped you along the way. Need help? Don’t be shy, we don’t bite!