The state of large language models – Where are we now? A business perspective
21 December 2023
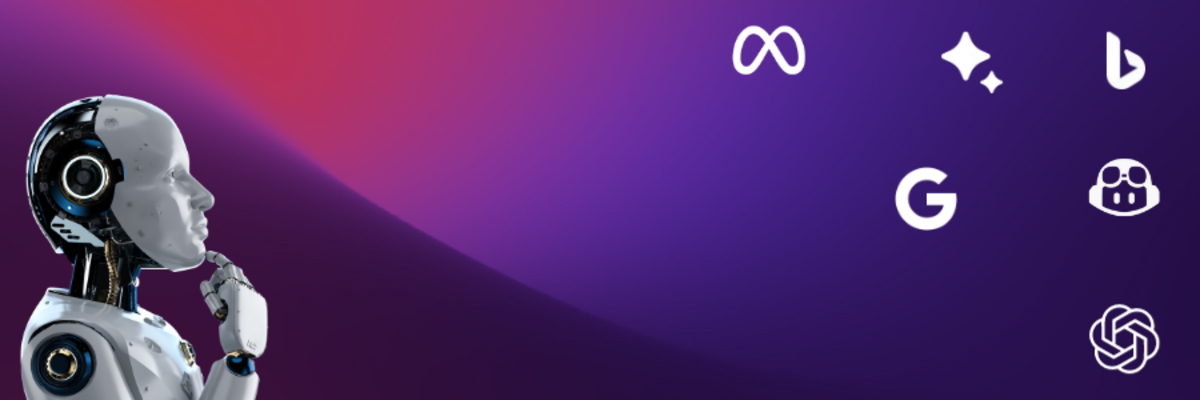
Just over a year ago, the ground-breaking introduction of chatGPT captivated the public... And captivated data professionals alike. Witnessing a computer program effortlessly comprehend natural language and respond to questions, left many of us astounded. Since the release of chatGPT, there has been a rapid surge in technological advancements in the field of Generative AI. Numerous large-scale AI models have emerged, some of which are even available as open source. The landscape evolved to encompass innovative AI applications like GitHub Copilot. Also, it expanded beyond mere text to include multimodal models capable of processing images, sound, and text seamlessly. Despite these strides, it is still difficult for businesses to successfully apply these new technologies to their processes and generate real value from them.
In this blogpost, we will delve into the technology of Large Language Models (LLMs), examine the diverse options to access this technology, and explore potential use cases. This summary aims to provide a comprehensive understanding of how these advancements can be applied to generate tangible business value.
Technological capabilities of Large Language Models
First things first, let’s look at this from a technological standpoint. These large language models showcase versatility by excelling in 4 distinct tasks:
- These models adeptly categorize various forms of text, ranging from extensive documents to concise paragraphs and individual words. For example, LLMs could classify emails in groups like, read now, read later, or don’t read.
- LLMs highlight proficiency in crafting coherent and logical textual completions. They excel not only in understanding context but also in generating contextually relevant content. An example is completing an email based on the first couple of lines.
- Training LLMs to respond to queries effectively, transforming them into fully-fledged chatbots like chatGPT.
- Leveraging LLMs as intelligent agents. In this role, LLMs serve as the cognitive power behind autonomous systems, allowing them to strategize, plan, and execute specific tasks. This multifaceted functionality underscores the expansive potential of LLMs across diverse applications and opens possibilities for fully digital assistants that can take meeting notes, summarize, and request feedback all based on a single prompt.
The fact that these models can perform these tasks is great, but the difficult application of this technology in the business context hints that there might still be some limitations. LLMs still have some open challenges regarding their technology, accuracy, and alignment.
Challenges and Limitations for LLMs
The application of LLMs unveils certain challenges and limitations from a technological perspective:
- The reliance on extensive data for training poses logistical hurdles in terms of movement, verification, and storage.
- Both the training and inference phases often entail prolonged durations, presenting practical constraints.
- The accuracy of LLMs, a pivotal concern for businesses, is shadowed by various complexities. Outdated training data can lead to models generating responses based on obsolete information.
- These models grapple with contextual limitations, restricting their ability to consider the full context, and thus the needed nuances, for predictions. Notably, a well-known challenge is the tendency of LLMs to "hallucinate," occasionally producing text that deviates from factual reality.
- Moreover, businesses encounter a formidable challenge in aligning LLMs with their specific goals. The potential bias in the model's output, coupled with its unpredictability and evaluation difficulties, raises concerns. This complexity makes it challenging for businesses to ensure that the output of LLMs aligns with their expectations and objectives.
Despite the challenges, the undeniable value of LLMs remains. So that prompts the inevitable and crucial question: ‘how can we, as a business, effectively access this technology?’ Fortunately, there are several avenues to explore.
How to access Large Language Models
In the realm of Large Language Models, the age-old dichotomy of buying vs building technology remains.
Choosing to buy involves acquiring an AI application from another company, providing a swift and straightforward solution to a specific problem without the need for additional development work. While this option offers predictability in costs, there's a crucial caveat — ensuring the solution precisely aligns with your needs. Moreover, the risk of the providing company going bankrupt poses a potential threat to the longevity of your solution.
For those seeking flexibility, the build options offer varying degrees of investment. The simplest integration involves utilizing proprietary models via API, where you construct your application but rely on another company for the AI capabilities. While this approach provides access to high-performing models at reasonable prices, it often lacks transparency in how the LLM output is generated and may limit control over the model's future development.
If control is paramount, self-hosting an open-source model becomes a compelling option. This entails relying on the open-source community to develop a downloadable model, enabling you to host and integrate that model with your own application. Though it demands a higher upfront investment, this approach grants greater control over customizing the LLM and manipulating its output according to your specifications.
The most resource-intensive option involves training your own LLM from scratch, a path typically too costly for most companies starting their AI journey due to the substantial resources required.
Now that we understand how to access LLMs and have a view on the diverse options available on the tasks they can perform. We can explore what they can mean for your business.
Potential business value of Large Language Models
Large Language Models hold theoretical promise in various business applications, yet it's essential to gauge their impact accurately. Research conducted by the Boston Consulting Group and Harvard underscores the potential of GPT-4 to enhance the performance of knowledge workers. However, they emphasize that some tasks are better performed without the help of GPT-4 even when people are trained to use this new technology.
One compelling application of LLMs in business is the enhancement of efficiency in analyzing extensive textual datasets. For example, financial and legal analysts can spend hours digging through multiple documents finding the relevant information. Likewise, software developers that are handed over a new project can require a significant amount of time understanding a new codebase.
LLMs could support this analysis by pinpointing relevant documents, paragraphs, and even specific words. Moreover, they possess the capability to answer questions based on the insights extracted from these documents. This could result in significant time savings.
Another avenue where LLMs can make a significant difference is in streamlining the retrieval of textual documents. In large organizations it can be difficult to get a concrete piece of information to answer your questions. LLMs support here by identifying documents with similar meanings, expanding the scope of document retrieval beyond mere keyword-based search. Integrating this capability with a chatbot creates a dynamic digital assistant. This assistant can not only answer questions but also provide contextual responses based on the relevant documents it identifies, offering a seamless and efficient information retrieval system. Thus, resulting in more informed employees that must spend less time on finding relevant information.
Third, LLMs can empower non-technical users accessing complex systems. For some users, writing SQL, python or accessing a complex ERP system might be too much to ask for. LLMs could be leveraged as a natural language interface bridging the gap between these users and the technical system. Users can explain their requirements in natural language and the AI model will execute the technical command. This results in a wider range of users that can successfully interact with technical systems.
Conclusion
While chatGPT has captured global attention, its substantial impact on business processes remains constrained by persistent technological challenges. Nonetheless, the potential applications are abundant and whether opting to buy or build an AI solution, the imperative is clear — start experimenting with Large Language Models (LLMs) now. The presence and impact of these models are poised to expand significantly.
For businesses contemplating their entry into the realm of AI, the advice is straightforward: start today! Companies must proactively engage with this transformative technology to stay ahead. It is crucial to identify a small yet impactful use case, this allows business to get familiar with the technology and develop the needed capabilities to use it effectively.
If uncertainty persists about where to start this journey, feel free to reach out. We’ll help you get started finding a first use case and making sure value is realized.