Geospatial Analytics: From Theory to Impact
18 June 2024
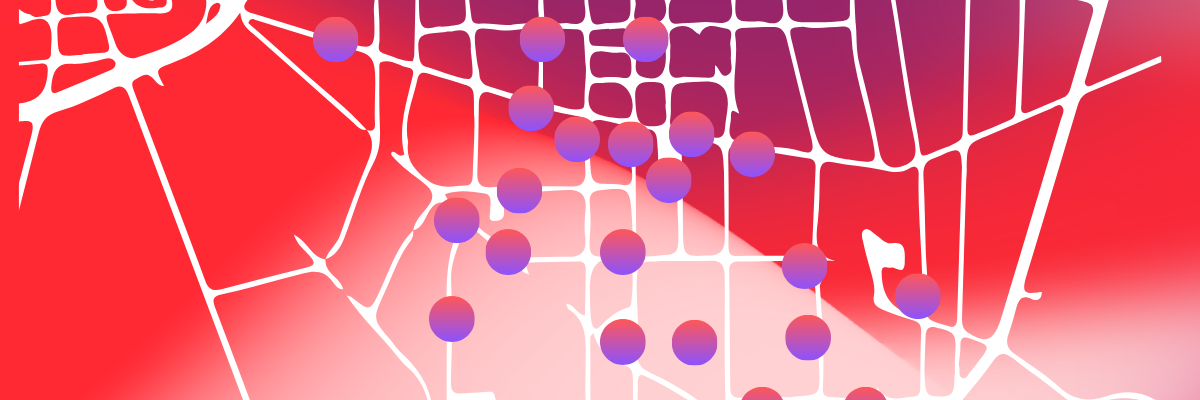
Geospatial analytics might be one of the most fun areas you could work on as a data professional, and one of the most useful as an end user. What makes it so enjoyable and useful is that it is a direct representation of the outside world. A nice variation in a world of orders, sales, customers, products, … It may be for this reason that geospatial data is the oldest data source in the history of human civilization. Indeed, the first map has been found engraved on a mammoth tusk dated to 25.000 BC, indicating our ancestors were data driven hunters.
Jokes aside, we can fast forward a bit to what we can consider the birth of modern spatial analytics. We are of course talking about the work Charles Picquet did in 1832 to investigate the cholera outbreak in Paris. He divided Paris into the 48 districts and coloured each one according to the death rate from cholera—ranging from lightest for fewest deaths to darkest for most. In modern terms this essentially translates to spatially joining points to polygons and aggregating by summation before finally visualizing the result on a map. This early use of a thematic map visually represented the varying intensity of the cholera outbreak across different areas, literally illustrating where the crisis was at its worst. While Picquet’s map was groundbreaking for its time as it provided a visual representation of data, it didn't necessarily spur immediate public health action aside from enabling city officials to prioritize hygienic interventions. That honour fell to a similar investigation performed by John Snow two decades later in London. This time the gained insights led to the discovery and subsequent closing of a contaminated water pump on Broad street.
![]() | ![]() |
Fast forwarding again to the birth of modern spatial analytics, we see geospatial data seamlessly integrated into our daily lives. Calling an uber? In the background a geospatial query is run to find the nearest available driver. Looking for your dream house on a real estate platform? Important insights such as price levels in the neighborhood, distance to parks and schools, … are considered. The possibilities are endless and in many cases truly useful.
In this post we give you an overview of the building blocks of this technology and its applications to help you spot opportunities where geospatial analytics could help your business.
Platforms & techniques in Geospatial Analytics
There are three main entry points to start levering this technology each with their own strengths and focus. Firstly, we have the traditional databases, or database extensions, which allows us to work with geospatial data right along traditional data and make it an equivalent data citizen in our data warehouse. We can leverage the same database structure with schemas, tables, columns, and write SQL statements to transform and join the data. The seamless integration of geospatial methods with your existing data and processes on the same platform makes this approach a very popular choice.
Sometimes you don’t have a platform at hand or your project is a one off. If you are comfortable with Python there is a whole ecosystem of packages out there for geospatial analytics. You could use Geopandas or Geospark which extend Pandas or Spark with geospatial capabilities. The flexibility provided by them make it ideal for power users and use cases with specific requirements.
Finally, it is also worth to mention there exists a whole class of GIS software suites. You might have heard of Arcgis or QGis. These packages are aimed more at GIS professionals and are less suited to perform geospatial analytics at scale, especially when you wish to integrate with your ETL. They are very good however at visualizing and editing geospatial data and can connect to your database to retrieve and store geospatial data.
Typical Applications of Geospatial Analytics
So once you’ve got your platform in place, where to begin?
Geospatial analytics starts with understanding its simplest components: points, lines, and polygons. Points mark specific locations on the map, like a bus stop or a coffee shop. Lines connect these dots and can represent routes, streets, or pipelines. Polygons are closed areas formed by lines, outlining regions such as city districts, lakes, or property boundaries. These shapes are the building blocks of geographic data analysis, representing concepts in utilites, emergency response planning, real estate management, conservation, and public policy decisions. Basic geospatial operations like measuring distances, calculating areas, or performing simple overlays are performed with these elements to transform raw data into actionable insights.
Once you've mastered basic shapes, you need to utilize advanced geospatial operations that layer additional insights onto this basic data framework. Operations like buffering, which creates a zone around a geographical feature, and spatial joining, which joins datasets based on their geographic relationship, allow for answering more complex problems. These advanced techniques help stakeholders make more informed and precise decisions based on spatial relationships and interactions.
Indeed, geospatial techniques aren’t just about maps. In the end it’s a strategic tool transforming how businesses makes critical decisions across various industries. For instance, understanding the potential impact of a new public transport system involves calculating how accessible it is from different points in the city. In the telecommunications sector, Belgian providers leverage these techniques identifying weak signal areas and mapping it on top of populations, thus strategically positioning new cell towers. Moreover, during mergers and acquisitions, geospatial insights allow firms to assess the geographical spread of assets and customer bases of potential targets, uncovering overlaps and synergies that can make or break the deal.
Use Cases of Geospatial Analytics
Dataset enrichment
When creating datasets, you probably use them for decision making in the end. Some may even be used as input for your machine learning models. Maybe it would be useful to incorporate geospatial features? It might be useful to know the distance to your nearest store for your customers and see how that influences their behaviour. Those insights can then be used as input when searching for an optimal location of the next store. Going even further, you might include the distance to your competitor’s nearest store. If your main concerns are not about customers, but buildings. In that case you could enrich your portfolio with insights such as flooding risk, social and demographic factors, and other relevant contexts to analyse and optimally manage your assets. Even though these basic enrichments are not great technical challenges, they have proven to be most useful.
Aggregating data
Collecting data where exactly things happened, like a transaction, event, or just the location of something stationary can be very valuable. However, chances are that putting all those datapoints on a map might not be very useful because of information overload. Many mapping components included in reporting platforms have a limit on the number of features they can show. Even if they manage and it might look cool, that level of detail limits is frequently not necessary. Doing some kind of geospatial aggregation beforehand can bring out more general and useful insights.
There are many approaches here. First of all, we can use something called H3 indexing which is a hierarchical geospatial index invented by Uber to analyse their ridesharing data. The system divides the globe into hexagons of different levels. Each hexagon is defined by an Id and is the parent of 7 hexagons on a lower level. There are many efficient functions to find the parent or children of a certain hexagon, or to find the hexagon of a specific level that contains a point. This makes it very easy and fast to aggregate data geospatially on different levels.
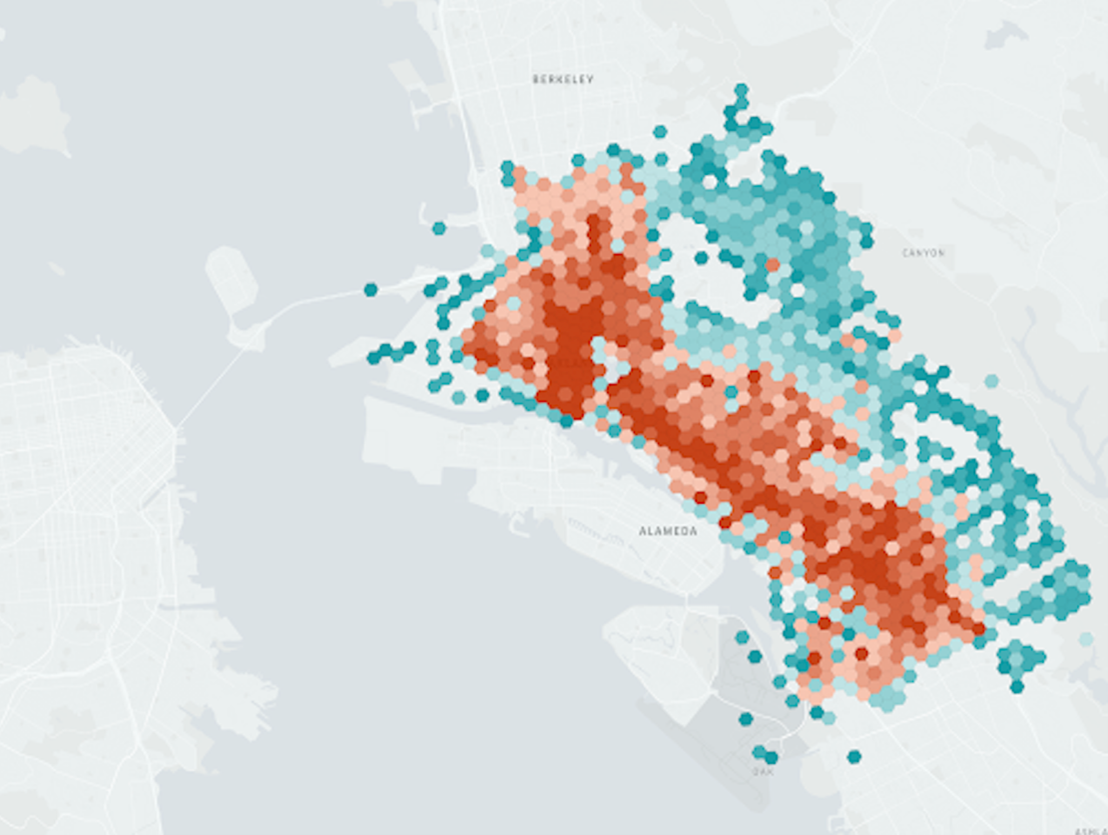
The other way we can aggregate data is using existing borders and places. In Belgium we can use what is called the AdminVector dataset. It is the dataset published by the government that contains all administrative units of the country: regions, provinces, arrondissements, municipalities, sub municipalities, and statistical sectors. They can be used to quickly answer questions like: how many properties do we have in Antwerp? In which neighbourhoods do we have a high (or low) proportion of customers? In which central cities do we have most of our deliveries? Because people know and understand the aggregation level from daily life, this can be most useful.
Fleet Management
In today's competitive logistics landscape, the integration of geospatial analysis into fleet management is essential for operational optimization. By using real-time GPS data, we can compare current fleet locations with scheduled routes and quickly forecast up to date estimates. This proactive approach not only reduces wait times but also boosts customer satisfaction. Additionally, analyzing speed patterns helps pinpoint recurring slowdowns, allowing for smarter route planning and scheduling. This leads to more efficient operations, lowered fuel consumption, and reduced maintenance costs. Utilizing historical geospatial data also supports predictive analytics, enabling businesses to anticipate and mitigate potential bottlenecks before they impact productivity. By adopting these geospatial strategies, companies position themselves for improved service delivery, cost efficiency, and sustainable growth.
Interested in what Datashift's broad expertise in analytics can do for your organisation? Do not hesitate to contact us and let's have a chat! We are always happy to listen to your data challenges and ambitions.