How to Leverage your Competitive Advantage in the Era of AI
27 June 2024
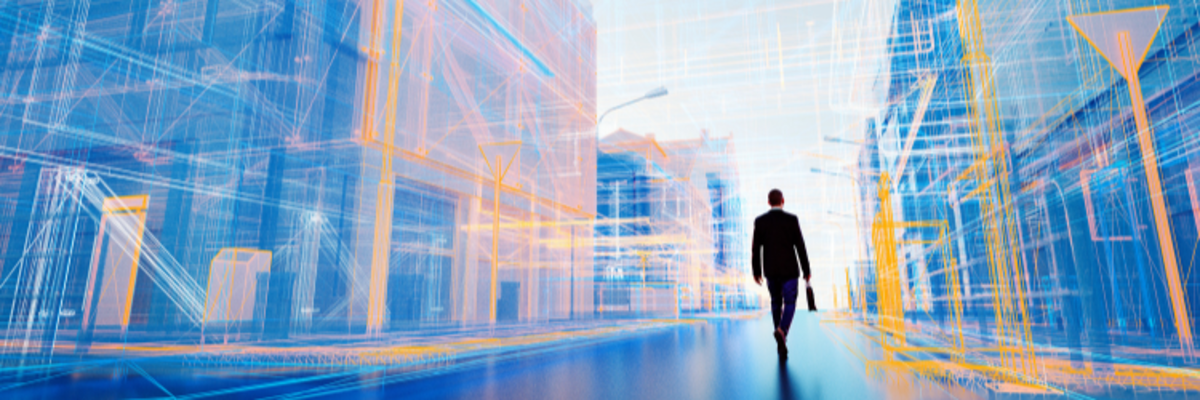
Artificial Intelligence, or better yet AI, is everywhere whether you like it or not. There is a lot of buzz around this topic but the reality is that the technology is exciting and incredible use-cases exist in every industry for every company. Those companies who will make the difference with AI will be the companies that reign successful in their industry. But how do you make the difference in AI when solution are available to all, either behind a paywall as a product or open-sourced to the whole community?
Of course you still need AI talent, a business model that is able to leverage the new capabilities, and strong integration between existing systems and new AI-pipelines. When you do this better than competition you can come out ahead. But you also have an option to make your solution unique by customizing your AI systems. An AI that is trained on your specific data cannot be copied by competitors and is a very strong way to set you apart. Let's have a look at how you can fine-tune your AI systems on your specific data.
What is fine tuning?
Fine-tuning leverages the vast knowledge embedded in pre-trained models to create solutions tailored to specific use cases. It involves starting with a pre-trained model, such as commercially available ones like GPT or open-source options like Llama. These models have already been trained on large sets of documents, enabling them to understand and generate language across various contexts.The fine-tuning process can begin with these general-purpose models or with ones already optimized for tasks like question-answering and instruction-following. However, these models might not yet produce outputs tailored to your specific business needs. Fine-tuning involves feeding the model with specific input-output pairs relevant to your domain, allowing it to learn the particular jargon, nuances, and styles required.Rather than adjusting all the model's parameters—which would demand immense computational resources—we typically adjust specific layers of parameters. This focused approach directs the model's output to align with the desired examples, achieving a balance between computational efficiency and the accuracy of the fine-tuned model.
Fine-tuning results in an specific and efficient model
After fine-tuning, the general model is transformed into a custom model that behaves in accordance with the specific data it was trained on, making it better suited to your unique needs. This technique enables the creation of models tailored to specific tasks, enhancing their relevance and effectiveness.Fine-tuning is also commonly used to develop efficient small language models. Large models require significant hardware resources and take longer to generate outputs. By scaling models down, hosting becomes easier and more cost-effective, but you pay with some loss in accuracy. However, fine-tuning these smaller models for specific tasks can enable them to perform on par with or even surpass their larger counterparts, delivering faster responses at a lower cost.
Fine-tuning in concrete use-cases
Fine-tuning a language model can be useful in many scenarios. Let’s explore a few. First, In the logistics sector, managing delivery notes manually is time-consuming and error-prone. A vision-language model can transform images of delivery notes into JSON files, making the data easy to integrate into IT systems. By default, language models output plain text, but for this task, we need JSON-specific text. Fine-tuning involves collecting input-output pairs where the input is an image of a delivery note and the output is the corresponding JSON. This process ensures the model generates accurate JSON representations of the delivery notes.
Secondly, In the legal field, language models can assist in drafting contracts. While general models can generate useful outputs, they can still produce a wide range of errors. Fine-tuning a model with examples of well-crafted contracts helps the model learn to produce higher-quality documents. This could lead to strengthening of a firm’s competitive advantage. Since they are able to leveraging their superior contract-drafting expertise and create a well-curated dataset to train a more effective virtual assistant.Third, In the healthcare industry, doctors and nurses working in remote or under-resourced areas often need decision support tools that can operate offline due to unreliable internet access. A fine-tuned language model can be hosted on mobile devices to provide medical advice, diagnose conditions, or suggest treatments based on patient symptoms and medical histories. By fine-tuning a model with a dataset of medical records and diagnostic guidelines, the mobile app can deliver accurate and reliable advice without needing internet connectivity. This ensures that healthcare professionals in remote locations have access to critical information, improving patient outcomes and operational efficiency.
Some challenges remain
While fine-tuning offers significant benefits and has become more accessible, several challenges remain:
- Data Quality: Data is essential for fine-tuning. The principle of "garbage in, garbage out" still applies, meaning the quality of your training data directly impacts the performance of the fine-tuned model. Obtaining high-quality training examples is a major challenge. Experimenting with creative uses of existing LLM solutions can help mitigate this issue to some extent.
- Access to GPUs: Fine-tuning requires substantial computational resources, particularly GPUs. It's not feasible to fine-tune large language models on a local laptop. Fortunately, cloud providers are making GPUs more accessible and reducing the need for significant upfront investments. Leveraging these cloud resources is crucial for successful fine-tuning.
- Time Investment: Fine-tuning a model takes time. Even ignoring the time spent on curating and validating training data, the process of feeding examples to the model, making predictions, and updating parameters can take hours or days. In fast-changing environments where frequent fine-tuning is necessary, automating your training pipeline becomes essential.
Fine-tuning is an increasingly accessible method to make models more specific, incorporate new data, and run smaller, more efficient models. However, data quality, governance, and a centralized platform remain critical components. Let's connect to explore how you can leverage AI to make a difference.
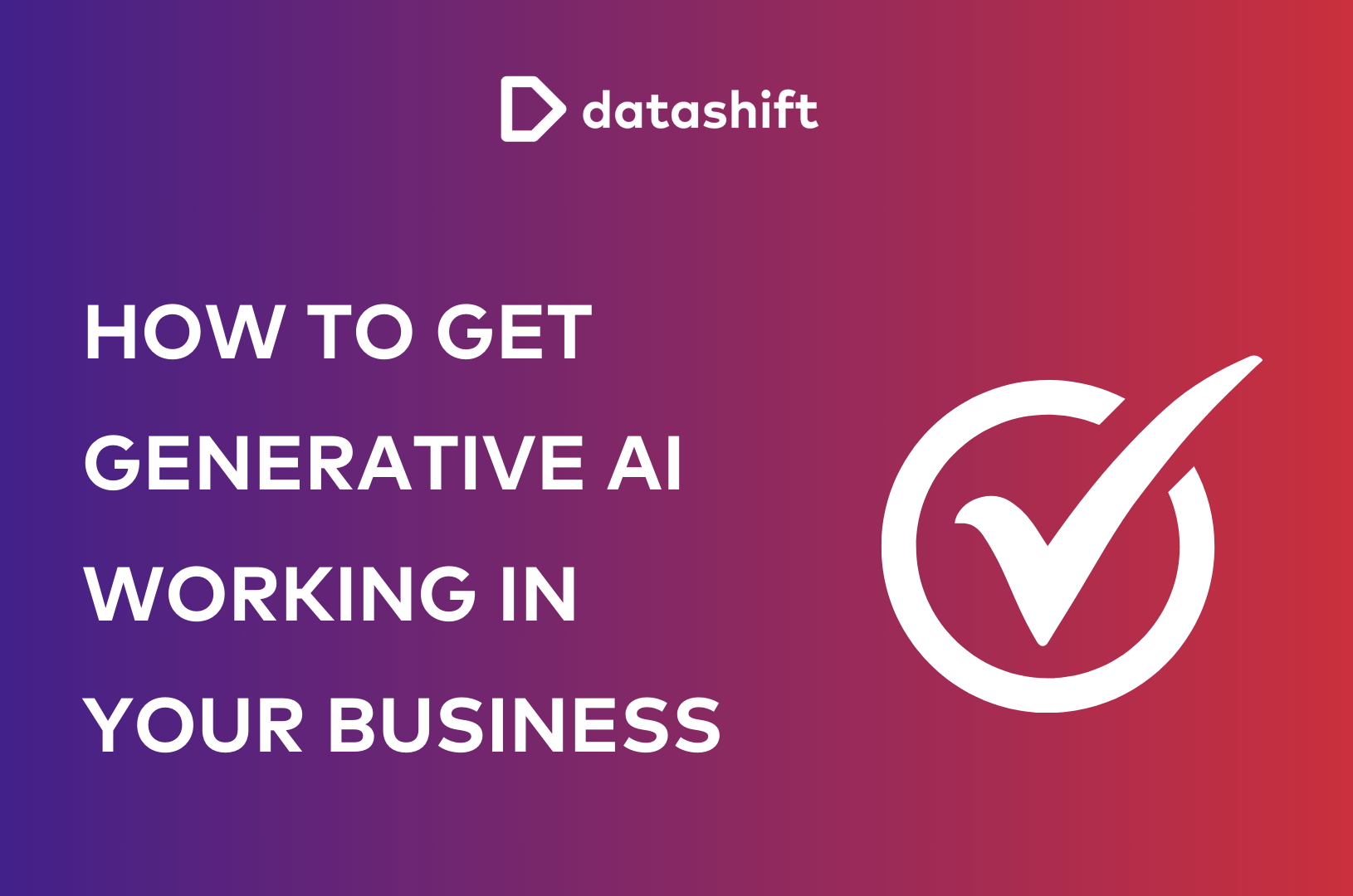