Semantic data discovery: separating facts from fairy tales
20 October 2022
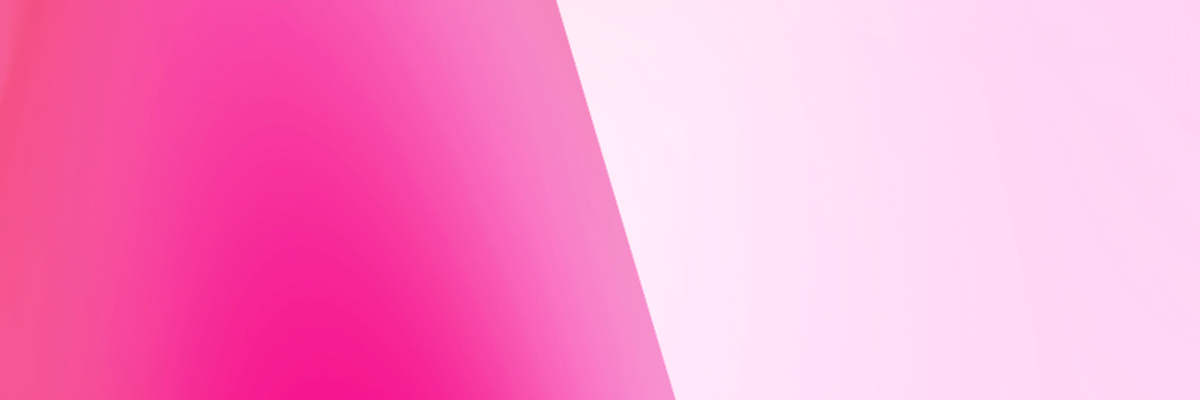
Do you have a team of business users and data specialists working closely to review and classify data files and tables by mapping business terms? That's great. But then, there are limits to this kind of manual approach. Not only does it take a lot of time, but it's also very likely that your team will overlook quite a few things.
Now, this is where semantic data discovery comes in.
The promises of semantic data discovery
Semantic data discovery is a process that helps you derive business meaning from your data in an automated way. It holds a two-fold promise: delivering a better understanding of your data (thereby improving data quality and making data governance easier) and facilitating the automation of your business processes.
The question, of course, is whether semantic data discovery can deliver on those promises. We know by experience that it can. However, that does not mean you can use it as a magic wand. In our experience, having expertise within your organization is crucial to success. In addition, you need to approach it with an open mind, staying focused on improving end-to-end processes.
So let's separate facts from fairy tales, making sure you understand what you can use semantic data discovery for, how it works, and what to look out for.
What you can use semantic data discovery for
Simply put, semantic data discovery helps you find the data you think you have somewhere, but you don't actually know where to find them. It can even help you unveil information that remained hidden so far in your applications and databases, and achieves that by extracting meaning from your data. It can do that because it takes the relevant terms for your data consumers as a starting point rather than the data itself.
Semantic data discovery saves time and increases efficiency by automatically mapping relevant business terms to your data tables and columns. Once that's accomplished, you can use the same tests to further improve the quality of your data sets by testing them against the properties they should possess. To what extent, for example, have national register number data been entered correctly?
And finally, semantic data discovery can also help you assess whether all personal data in your applications and databases has been correctly identified as sensitive data under GDPR. And that works in two directions: you can detect undeclared GDPR data as well as data that has been wrongly classified as sensitive simply because your data specialists wanted to be on the safe side.
How semantic data discovery works
Now that we’ve covered some typical examples of what you can use semantic data discovery for, let's dive deeper into how tools such as Ab Initio, Microsoft Purview, BigID, or Collibra work.
In the first step, data profiling processes collect descriptive statistics (such as data types, min/max values, and recurring patterns) about the data stored in your data platform. In other words: those processes extract the essence of your source data.
Next, a series of discovery tests combine those data profiles with business terms and reference tables to provide your data governance platform with suggestions on how your source data relate to your business terms:
- Tests that scan the headers of your data columns for useful information.
- Pattern tests verify whether the data conform to a specific pattern (an IBAN or a phone number, for example).
- Reference list tests examine if specific entries on a list of first names, family names, countries, family relationships, … can be found in data fields.
- Tests that inspect if parts of data fields match with entries on a reference list of street suffixes, job titles, ...
Afterward, fine-tuning is needed to override for words such as “NA” and clean up the results of the former tests. The results of all this are then aggregated to accurately predict what your data columns stand for.
Finally, human judgment is required to interpret the outcome of those discovery tests and, if deemed to be correct, confirm and feed them into your data governance platform.
What to look out for with semantic data discovery
The process we've just described transcends the mere use of a semantic data discovery tool. While those tools are indispensable to achieving what you're looking for, they can only do as much as you ask them to do. In other words, you need to specify the business terms from which those tools should start working. So don't start from what you know to be in your data tables (or what you believe to be there) because your data discovery tests will simply overfit your data. Instead, approach semantic data discovery from a business perspective, staying 100% focused on identifying relevant business terms and improving end-to-end processes.
As we mentioned before, having the necessary expertise within your organization is crucial to success. That is especially the case because of the many challenges that come with discovery testing. For example, tests need to consider where more context can be found around specific data, such as the differentiation between professional and private addresses. Also, requirements need to be gathered for every business term to enable the development of tests that provide accurate results and are specific to your organization. All this boils down to having the business knowledge required to make your semantic data discovery tool do what you want.
Curious to hear more?
If you start with that mindset, then semantic data discovery can deliver on its two-fold promise of providing a better understanding of your data and facilitating the automation of your business processes. The best proof: in one of our recent projects, we identified a meaningful number of additional data elements that our client had not even considered yet!
Impressed (like our client was) and curious to hear more? Let us hear from you.